This results in more innovation, competitiveness, and development in the fast-changing digital world. Tools for drift detection and alerts ensure models function at their greatest. Data preparation is time-intensive, highlighting the need for effective model lifecycle management information handling approaches.
Deciphering: How Do Shoppers Make Buy Decisions?
Deploy machine learning in your organisations effectively and effectively. Are you trying to https://www.globalcloudteam.com/ implement Model Lifecycle Management or exploring ways to deliver effectivity and governance to your AI & ML? Look for extra articles coming quickly in our MLM Series or join an upcoming discussion.
Process Analytical Expertise (pat) Mannequin Lifecycle Management
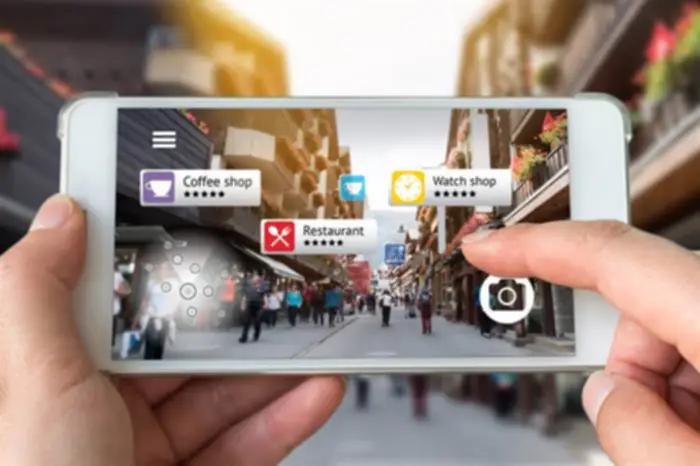
With the growing want for AI purposes, scalability is paramount. This entails evaluating and optimizing infrastructure for environment friendly model use. Given the computational prices of model coaching, entry to advanced resources and distributed computing is usually needed. Understanding how refined AI techniques reach conclusions is arduous.
What Instruments And Technologies Assist Ai Mannequin Lifecycle Management?
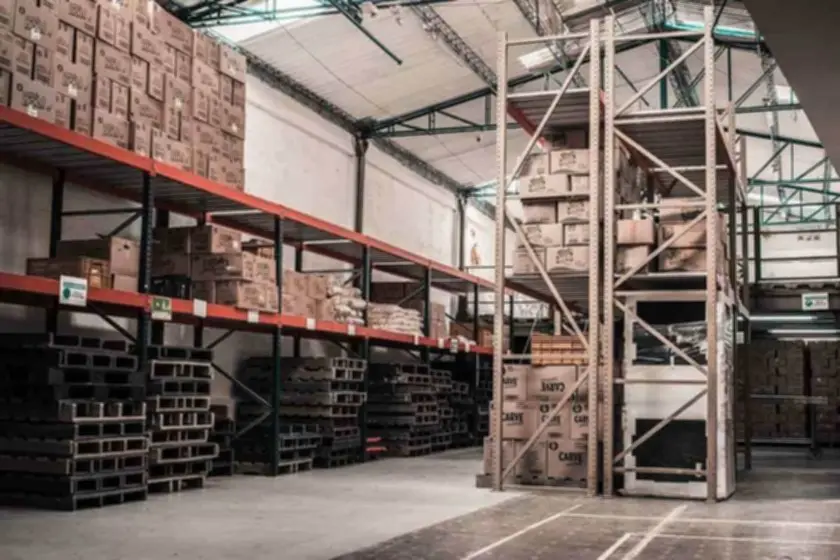
Whatever the type of machine studying mannequin chosen, preliminary exploratory analysis of the information ought to be carried out by an information specialist. Exploratory techniques may help knowledge scientists perceive primary options of the info, such as its basic features or groupings. This informs each the preparation of the info and the configuration of human-controlled elements like hyperparameters. It is simply via iterative fine-tuning that the mannequin is honed to supply the desired outcome. Learn extra about types of machine learning and fashions in Chapter 1. The AI lifecycle is the iterative means of transferring from a business problem to an AI solution that solves that drawback.
Embrace Mannequin Lifecycle Management To Tackle The Challenges Of Doing Ml At Scale
Throughout the MLC Process, you'll find a way to embody User Tasks to direct specific team members or roles to evaluation and approve adjustments to the fashions. ModelOp Center integrates with existing IT task administration methods, similar to JIRA, and ticketing systems, such as ServiceNow to include these person primarily based duties. For each of those externally-created duties or approvals, the MLC can inject model-specific metadata to supply context for the task or approval approval.
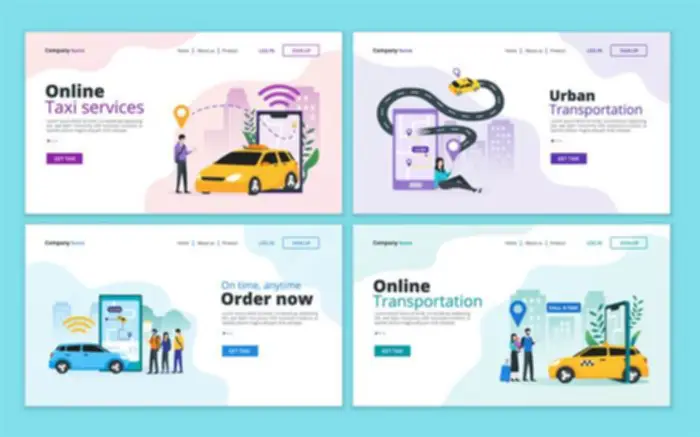
Promote A Mannequin Across Environments
It smoothes development and deployment steps, enhances team collaboration, and ensures model repairs. Adopting MLOps leads to optimized AI initiatives, unlocking their full worth and supporting long-term enterprise development. The reliability and efficiency of AI models are key advantages of lifecycle management. Creating a strong foundation for creating, evaluating, and refining models results in constant and exact outcomes.
Working A Enterprise Better: Cognitive Process Automation (cpa)
These embody each pre-processing and what kind of model should be used. In the Trikafta example, NIR is used for ultimate mix potency, and the data bear three pretreatment steps, with each step narrowing the spectral vary of interest. Our joint strategy can help clients with the whole data model lifecycle – a steady strategy of updating, reviewing, decision-making, and analysis. Model aliases permit you to assign a mutable, named reference to a selected version of a registered mannequin. You can use aliases to indicate the deployment standing of a mannequin version. For example, you could allocate a “Champion” alias to the model model at present in manufacturing and goal this alias in workloads that use the production mannequin.
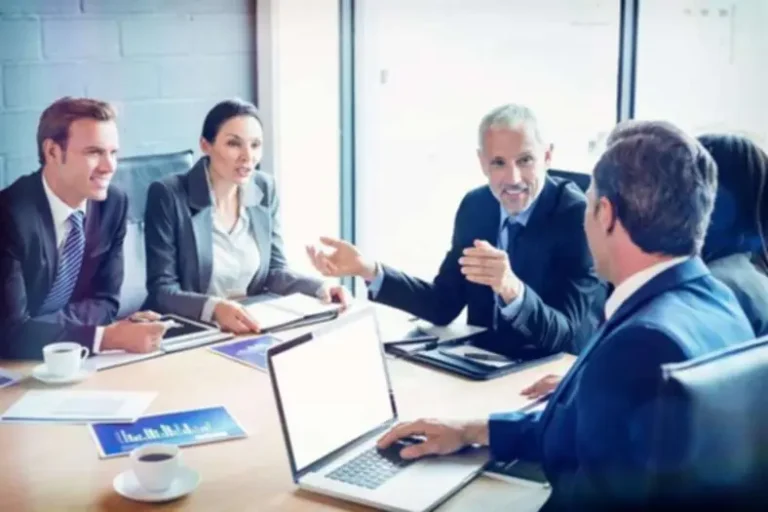
- The example in Deploy with Test and Jira includes these deployment pieces.
- Tools for drift detection and alerts ensure fashions operate at their best.
- Setting up strong governance and moral practices is necessary too.
- This bridges the knowledge and interest gaps between information science and operations groups.
- Automation might help streamline these processes, but it’s crucial that this technology is carried out in a method that still allows for efficient human oversight.
Lorem Ipsum is just dummy text of the printing and typesetting industry. Lorem Ipsum has been the industry's standard dummy text ever for the reason that 1500s, when an unknown printer took a galley of sort and scrambled it to make a kind specimen book. It has survived not only 5 centuries, but also the leap into electronic typesetting, remaining primarily unchanged.
This opacity can erode trust and create compliance risks, particularly in regulated sectors. To overcome this, investing in instruments for model interpretability, like characteristic significance evaluation, is vital. Finally, each time enhancements or modifications are necessary for an already productionized model, the mannequin enters the same lifecycle course of once more. At this stage, the second line of defence analyzes all documentation that has been submitted until this moment. If the unbiased evaluation is successful, the mannequin life cycle process moves to stage 5 – Approval. If issues are detected, the method is moved again to the first line of defence where further data needs to be generated.
For example, supervised machine studying fashions require labelled datasets to be taught the connection between input and output information. Labelled information must usually be ready by an information scientist, which is a labour-intensive process. Unsupervised machine learning then again is often used for figuring out trends and patterns in datasets. It will be taught from unlabelled data sets which can often require less preliminary preparation compared to labelled knowledge. A typical model improvement process includes identifying factors/drivers, data searching, cleaning and transformation, improvement, validation – business & statistical and at last productionisation.
They make it easy for knowledge consultants and builders to wrangle various datasets, take a look at variant models, and trace again through the historical past of changes. With this growth, corporations are realizing the need for a strong technique in dealing with their AI models. This approach, known as AI model lifecycle management, helps businesses successfully work with their AI. It merges the fields of information science and software engineering, permitting for higher creation, deployment, and management of AI techniques.
The fourth step is to watch the AI models in the manufacturing setting, where they'll face real-world situations and challenges. Model monitoring includes amassing, analyzing, and reporting the model performance, conduct, and suggestions, and detecting any anomalies, errors, or drifts. You ought to observe some model monitoring standards, corresponding to utilizing metrics, dashboards, and alerts, and documenting the mannequin outcomes, impacts, and points.